Can you spot when your data goes against the flow?
Anomaly Detection can be applied to operational monitoring metrics or business metrics that support decision-making right across the travel ecosystem and beyond. We discuss here how detecting small deviations in your traffic data can make a big difference to your revenue potential.
More and more data is flowing through our systems with our ability to collect and store it attempting to keep up with the flow. But our capacity to consume all this data and mine it for critical insights is being challenged. We have our human limits and need business intelligence (BI) tools to help us see our KPIs more clearly. Today AI is being introduced to complement BI in this endeavour. The aim is to help humans concentrate on using the data rather than the tasks involved in getting it.
These limitations can result in missed opportunities to discern patterns, identify events and their causes, and take corrective actions, especially at a time when unexpected business incidents occur. Large datasets have become difficult to manage as organisations are needing to make faster decisions in real-time. Anomaly detection using AI techniques is emerging as one of the critical technologies that organisations are leveraging to overcome this challenge.
What is an Anomaly?
In the time and price sensitive world of selling travel products, things inevitably can change or go wrong and there is an imperative to sound the alarm and identify the fix as soon as possible. To do this, the issue needs to be identified. This is where the ability to detect data anomalies early becomes a business differentiator.
An “anomaly” is simply a deviation from what is standard, normal, or expected and Anomaly Detection is the identification of atypical patterns in large data sets. An easy to understand example is your bank identifying out-of-the-ordinary purchases as fraudulent. But in practice, anomaly detection is more complex and nuanced which can make it daunting, although critical for organisations to implement.
Why Detecting Anomalies is important?
For travel product suppliers (such as hotels, airlines and intermediaries) selling inventory through API connections and websites makes their business success increasingly reliant on connectivity between a myriad of electronic platforms. Every room, seat or service sold involves high volumes of search data and transactions related to bookings. So early detection of events that could cause a negative impact to this flow of transactions (i.e. conversions and sales) becomes imperative. For businesses running thousands, millions, or even billions of dollars’ worth of transactions every day, the impact of this can’t be overstated. Time is definitely money.
How Detection Works
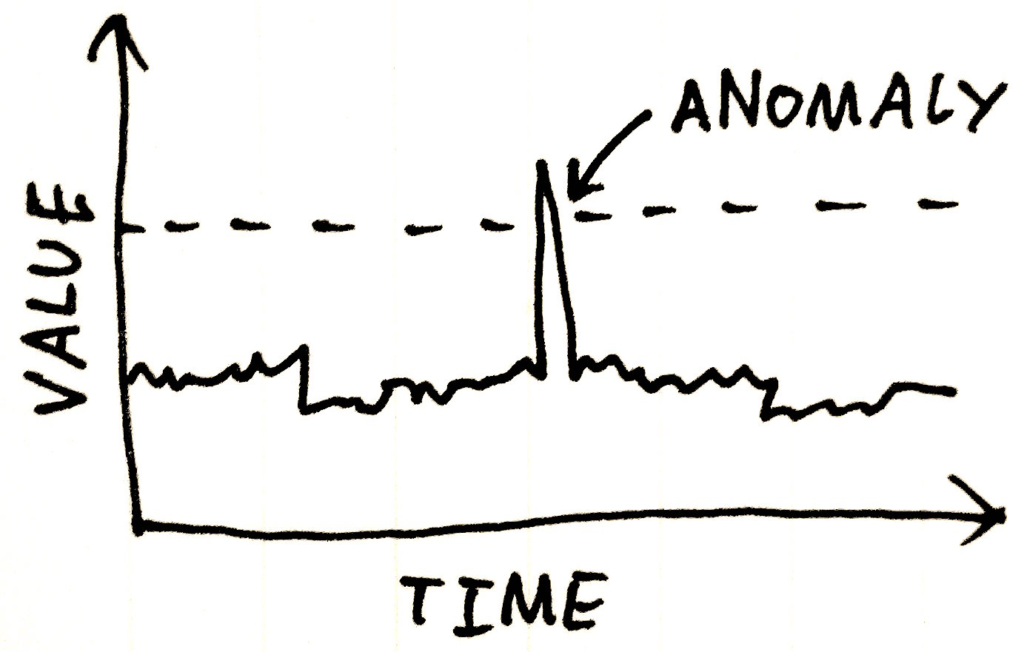
Detecting changes in time series data has a wide applicability. In a complex time-series data environment (which is what most travel transactions are), the detection tool uses a mix of rule-based checks, and machine learning algorithms to determine the parameters of normal behaviour and the instances when ‘normal’ is breached. This breach of expectation is highlighted as an anomaly. The detector looks at historical data, calculates anomaly points and presents the information visually in a separate Anomaly Detection data view. And all this, while accumulating more intelligence for the next time.
At the practical level, patterns can be identified to reflect the norms of doing business over given periods, be it daily, weekly, or monthly. Anomaly detection should then be able to absorb these patterns and uncover the unusual, rather than the norm. Since booking patterns can change with the introduction of deals, or events, or seasonal fluctuations, the detection algorithms quickly need to be able to learn the new patterns and adapt the detection profile.
Automated, real-time anomaly detection platforms can delve deep into data to pinpoint anomalies that wouldn’t be noticed by a human user monitoring datasets on a dashboard with subtle but potentially costly implications. The more traditional method of setting thresholds on KPIs to trigger alerts when the numbers go either too high or too low is proving less efficient when compared to the efficacy of machine-learning systems.
That said, anomaly detection can be difficult to get right at first and a pitfall to bear in mind is that complex data may sometimes result in false positives or negatives, i.e. an incorrect reading for what is really happening. Evaluating anomaly detection is a particularly fine balance. False negatives can be detrimental, but too many false positives can also render the system useless, or impact reputations. Real time systems don’t allow for second review of any potential anomalies so here there is a need for as much accuracy as possible in the detecting models used. Think of the example, where your bank is constantly blocking your funds due to false positives.
The solution here is to spend the time upfront on the detection engineering to make sure not too many false negatives or positives occur and to ensure learning, refinements and improvements continue, even after the model is in production.
How Travel Organisations Can Benefit from Anomaly Detection
Anomaly detection can be set for any KPI metric, and there are many business functions that stand to benefit from this innovation. Anomalies don’t always have to indicate that something is wrong. They can also be used to detect or predict slight changes in customer behaviour that could be translated into a shift in the way a product is marketed or sold. Basically insights to help businesses stay a step ahead of new trends.
Wherever critical KPIs are measured, Anomaly Detection can be used to dig deeper and see further. Some of the most valuable metrics organisations wish to monitor can also be the most variable. Application throughput, search requests and replies, response times and timeouts are all important metrics with pronounced peaks and valleys, depending on the time of day or the day of the week. Accurate detection can go a long way to successfully predicting potential failures or opportunities. Below are just three examples where Anomaly Detection could be used:
- Traffic dropped or spiked: Detect unusual changes to the normal levels of traffic, which might not be steady over the year. Anomaly Detection can determine what the normal traffic pattern is and flag an anomaly when traffic deviates from that norm.
- When bookings or revenue drop off: Anomaly Detection can determine the normal pattern for transactions or revenue and flag an anomaly when they drop below that norm.
- Scraping attacks: In travel searches, anomalous pattern detection combined with deep traffic analysis, can help identify instances of excessive price scraping by competitors. Detecting and mitigating malicious bots that scrape pricing data and unique content could help protect travel business against pricing and content leakage.
In summary, Anomaly Detection can be applied to operational monitoring metrics or business metrics that support decision-making right across the travel ecosystem and beyond. The unifying factor is the ability to detect small changes or differences in system or data message flow that might otherwise go unnoticed. Uncovering anomalies using machine learning allows humans (or indeed other automated systems) to take action based on these deviations. Depending on the circumstance, it is this action taking that saves costs, avoids losses and/or improves revenues.